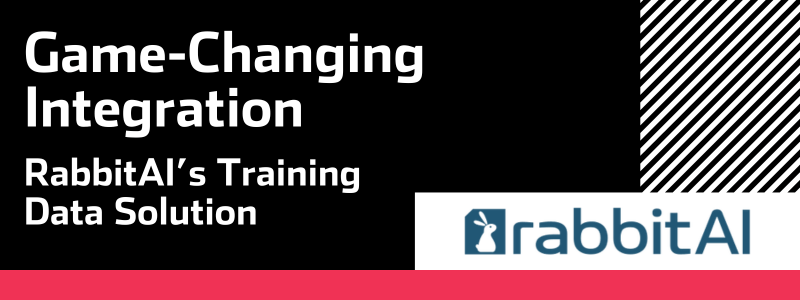
In the ever-evolving world of computer vision, the quality of training data plays a pivotal role in the success of AI models. RabbitAI is at the forefront of this revolution, offering a groundbreaking solution that seamlessly integrates synthetic and real data. By blurring the lines between what is traditionally considered “real” and “synthetic,” RabbitAI enables a flexible approach to training data that meets the unique needs of its customers.
This editorial explores how RabbitAI’s innovative methods are transforming the way training data is gathered, processed, and applied, ultimately providing unmatched value to its clients.
You call your training data “game changing”. What exactly has this effect?
When talking about the best training data for computer vision, there is one particular question that comes up again and again: Is real and synthetic data better? Our answer is: both are actually the same.
On the one hand, you can put a lot of effort into a realistic-looking synthetic image. Sometimes, however, this effort is simply too much.
On the other hand, when you take a photo in the real world with a camera, it’s actually digital. It becomes a mathematical matrix. This also makes it ‘synthetic’, in a sense.
So what is really real anyway? We want to show that this dichotomy does not exist or does not have to exist. With our method, you can slide from the synthetic to the realistic part of your recordings like a slider. According to the requirements of your training data. And this can indeed be an incredible game changer when it comes to gathering training data.
How does this solution benefit your customers?
Some scenarios can be captured in the real world more easily, while others can be modelled more efficiently. Then there’s the human X-factor. We only know to a limited extent how people will behave. Therefore, we cannot assess the extent to which a certain human behavior is indicative of drowsiness or another impairment, for example. We can only obtain a reliable reference by measuring this.
So if we want to reconstruct edge cases to test whether the algorithm can cope with things like sunglasses that occlude the eyes, for example, this is a situation that we can enrich synthetically. The rotation of the head, for example, has already been computed. This situation can therefore be extended quite easily to include sunglasses. The advantage for our customers here is that they can use a scene that has already been shot many multiples times, as there are almost no limits to the expansion of synthetic components. In addition, we are also able to adapt the camera hardware to various other specifications. In other words, our customer has the advantage of avoiding having to reshoot the scenes if a new camera setup is required. This saves them a lot of time as well as enormous costs, as they receive a huge amount of training data in a very short space of time.
Where does the rabbitAI solution make the most impact?
In fact, our solution is perfect for everything that falls into the area of monitoring safety-relevant systems. Take, for example, the correct triggering of an airbag. If this system has not been trained with different body postures, it can cause serious injury even though it is supposed to provide safety.
Our understanding of the situation as a whole is a decisive advantage here. For example, we know that OEMs need to make sure that the installed hardware works perfectly with the safety specifications and has therefore been trained with real, validated recordings. We also understand that TIER1 suppliers need to prepare their systems with the greatest possible variance of real-world training data. We therefore offer both ‘sides’ the safety of validated data as well as the high variance through enrichment with synthetic data.
How long does it take to integrate the training data into an existing development cycle?
Of course, we usually start by asking the customer what exactly they want to achieve and what their requirements are. Then we start to create a prototype. This takes between 2 and 4 weeks on average. We then build a reference rig. This takes about 1-2 months. In total, it takes a maximum of 3 months.
If required, we can derive synthetic data and support the iteration of the development cycles.
RabbitAI’s approach to training data is more than just innovative; it’s transformative. By offering the ability to combine real and synthetic data seamlessly, RabbitAI provides its clients with a powerful tool that not only saves time and costs but also enhances the safety and reliability of AI systems. Whether it’s optimizing the deployment of airbags in vehicles or refining the behavior of monitoring systems, RabbitAI ensures that every aspect of the process is fine-tuned to perfection. As the landscape of AI continues to evolve, RabbitAI stands as a beacon of progress, driving the industry forward with its game-changing solutions.